Teaching in courses:
Research Group
Biomedical Signal Processing
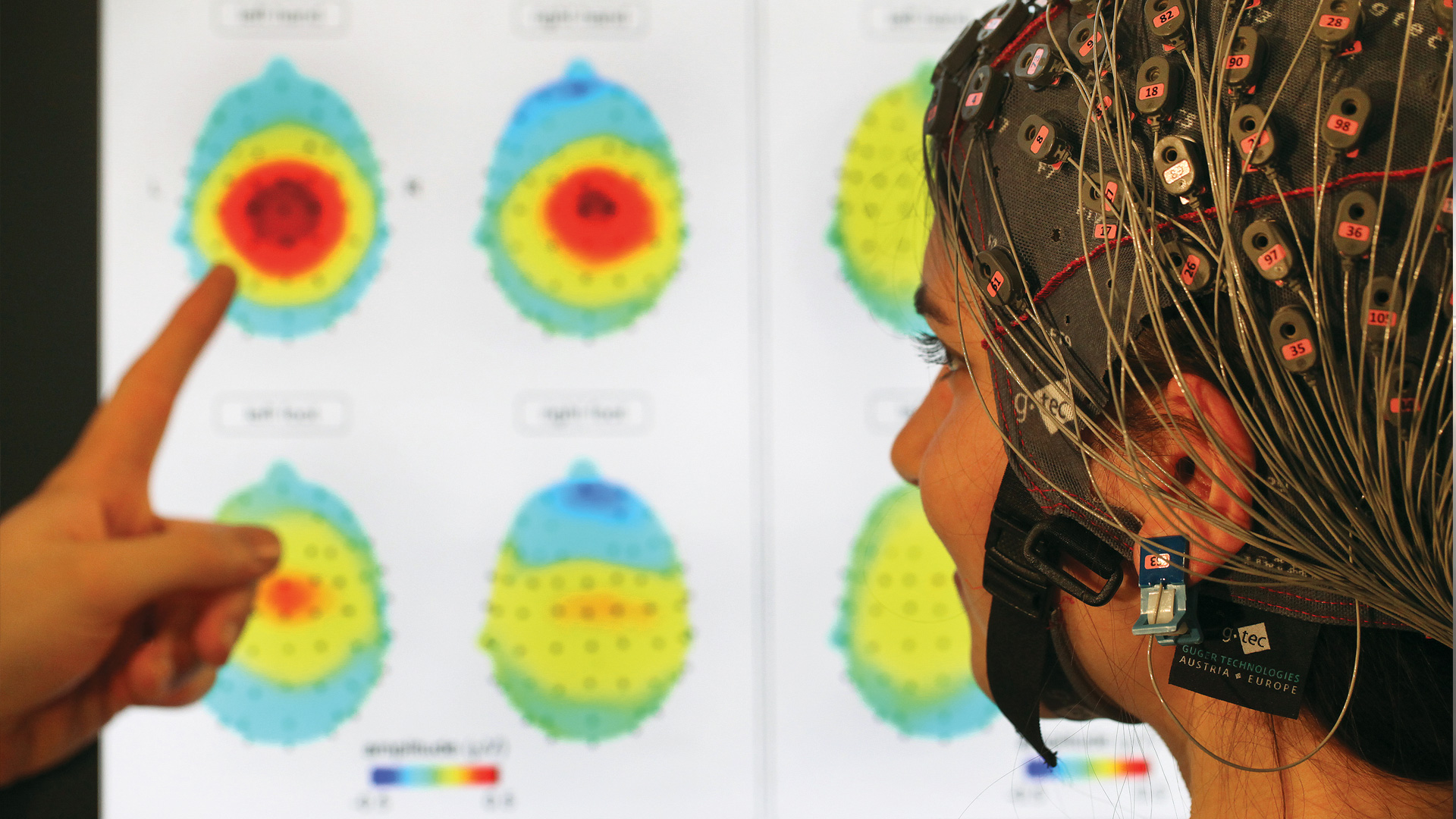
Group leader: Sadasivan Puthusserypady
Biomedical Signal Processing (BSP) is an interdisciplinary field merging engineering, computer science, and healthcare. It focuses on extracting valuable information from physiological signals like ECGs, EEGs, EMGs, and medical imaging data. BSP aims to enhance medical research, clinical practice, and healthcare technologies by utilizing signal processing algorithms, statistical analysis, machine learning (ML), and artificial intelligence (AI). It plays a vital role in diagnosis, monitoring, and treatment of medical conditions. In our BSP group, we research two exciting areas in digital health: (i) Brain-Computer Interface (BCI) for clinical applications, and (ii) AI techniques for cardiac health.
BCI enables direct communication between the brain and external devices, benefiting neurological rehabilitation and assistive technologies. Applying BCI in clinical settings improves the lives of individuals with neurological conditions. AI techniques in cardiac health facilitate early detection, personalized treatment, and preventive care. By analyzing vast amounts of cardiac data, AI identifies abnormalities and patterns indicative of cardiac conditions. These research areas merge technology with clinical applications to advance healthcare, improve patient outcomes, and enhance overall well-being.

BCI for clinical applications
BCI technology revolutionizes clinical applications in healthcare by enabling direct brain-to-device communication. It offers innovative solutions for individuals with neurological conditions, addressing paralysis, motor impairments, and communication disorders. BCI finds applications in neurorehabilitation, assistive technologies, and cognitive neuroscience. It controls robotic prosthetics, restores motor function, enables thought-based communication, and aids in diagnosing and treating neurological disorders. With signal processing advancements, AI, and real-time data analysis, BCI facilitates personalized and adaptive healthcare interventions. Ongoing research and collaboration promise to enhance patient care and push the boundaries of medical science, improving the lives of individuals with neurological conditions.
Ongoing research projects:
BCI for stroke rehabilitation:
Stroke is a debilitating condition that often requires extensive rehabilitation to restore lost motor and cognitive functions. In recent years, the field of healthcare has witnessed the emergence of innovative approaches combining brain-computer interface (BCI) technology, artificial intelligence (AI), and machine learning to revolutionize stroke rehabilitation. BCIs enable direct communication between the brain and external devices, providing a pathway for individuals to control assistive devices or prosthetics using their thoughts. By incorporating AI and machine learning algorithms, these systems can adapt and learn from neural signals to enhance stroke rehabilitation outcomes. AI-driven techniques analyze brain activity patterns, decode intentions, and customize rehabilitation programs to meet individual needs. This integration of BCI, AI, machine learning, and healthcare holds immense potential for improving the quality of life for stroke survivors by promoting neuroplasticity, optimizing rehabilitation protocols, and fostering personalized treatment plans. Through ongoing research and collaboration, these technologies continue to advance, offering new avenues for stroke rehabilitation and reshaping the future of healthcare.
- SmartRehab: Design and Development of Brain-Controlled Smart and Portable Rehabilitation Kit for Home-Based Stroke Recovery (2022-2026, Stanford University (USA), DTU Health Tech and Aarhus University, Funded By: Novo Nordisk Foundation, Denmark)
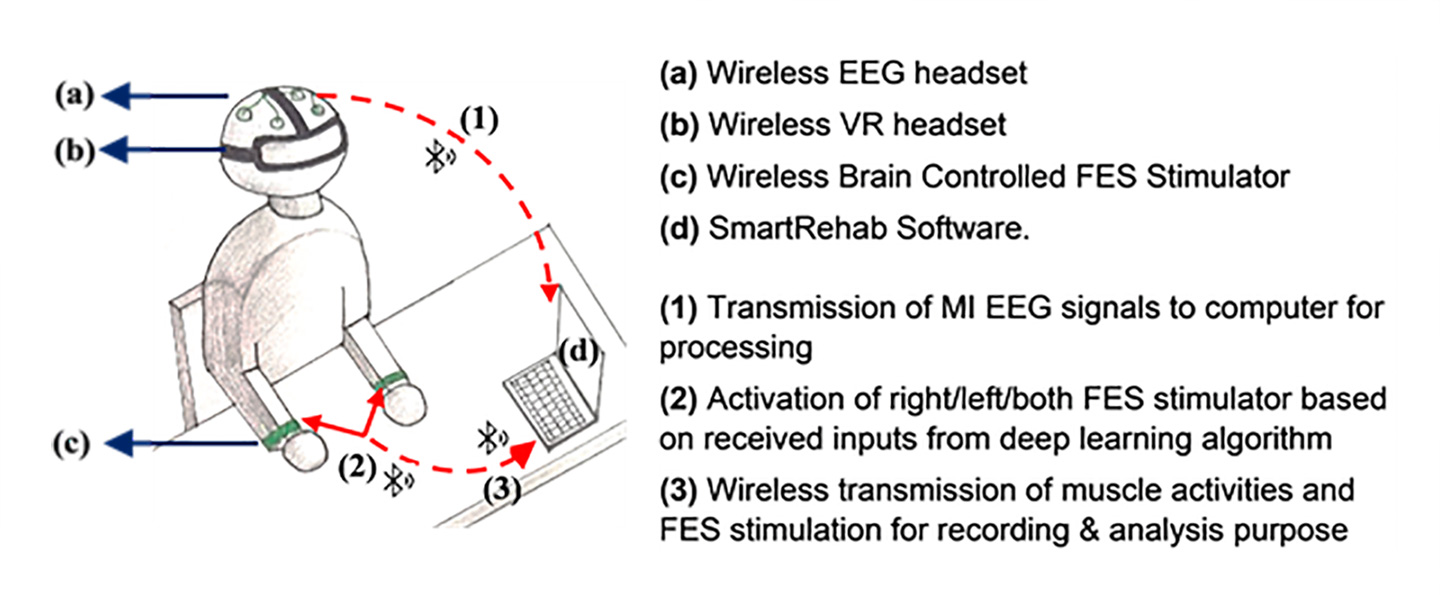
In SmartRehab, our goal is to develop a portable and wireless BCI Controlled Home-based Rehabilitation Kit for stroke survivors in the chronic phase. This kit focuses on upper limb rehabilitation and utilizes motor imagery and functional electrical stimulation (FES). In post-stroke paralysis, patients struggle to transmit signals from their motor cortex to their muscles. To restore movement, electrical stimulation is an effective method, but it is usually manually administered by therapists, limiting the patient's active involvement. Our solution utilizes motor imagery-based BCI systems, allowing patients to control the stimulation device using their brain signals. This promotes effective training and neural plasticity (brain network recovery). Unlike existing stroke rehabilitation systems limited to clinical settings, our proposed system overcomes these limitations by being completely portable and wireless. The project involves developing signal processing algorithms, creating a hardware prototype of the electrical muscle stimulator, and designing software modules. We will also conduct clinical pilot testing on stroke survivors. This project aims to provide a novel solution to health challenges associated with neural disabilities, making a significant societal impact.
- Command Generation for External Device Control via BCI and AI (2020-2023, Funded By: The Republic of Turkey Ministry of National Education)
EEG-based BCI systems utilize the subject's EEG signals to control external devices. The advancements in this research field have generated significant interest among researchers and students who seek to develop BCI schemes for various applications. To meet this demand, this PhD project aims to develop an AI-powered BCI signal analysis framework and toolset that enhances the usability of BCI systems in healthcare, smart environments, and communication. The primary objective is to create a user-friendly Deep Learning (DL) framework and accompanying guidance specifically designed for non-computer science researchers. This framework will facilitate the construction of a decision network for BCI systems, enabling the extraction of user commands from brain signals with minimal training effort. The generated toolset is expected to significantly reduce development efforts for BCI applications by minimizing the requirement for expert knowledge in BCI signal analysis.
AI techniques for cardiac health
Cardiac health is a critical aspect of overall well-being, and the early detection and management of cardiac arrhythmias play a vital role in maintaining a healthy heart. In the ever-evolving landscape of healthcare, the convergence of data science, artificial intelligence (AI), and arrhythmia detection has revolutionized the way we approach patient care. Data science, with its focus on extracting insights and knowledge from large datasets, has become a powerful tool in healthcare. AI techniques, such as machine learning and deep learning, have demonstrated remarkable capabilities in analyzing complex patterns and making accurate predictions. When applied to arrhythmia detection using ECG signals, these technologies offer unprecedented opportunities for early diagnosis, personalized treatment, and improved patient outcomes. By leveraging advanced algorithms and sophisticated models, healthcare providers can harness the potential of data-driven approaches to detect, classify, and monitor cardiac arrhythmias with enhanced accuracy and efficiency. This transformative synergy between data science, AI, and arrhythmia detection holds tremendous promise in reshaping the future of healthcare, paving the way for more precise, proactive, and patient-centric approaches to cardiovascular health.
Ongoing research projects:
- Digital Biomarker Identification for Stroke and Heart Failure (2022 – 2026, CATCH – The Cardio-relay Model for Cross-sectoral Ambulatory Treatment of Congestive Heart Disease based on Personal Health Technology, DTU HealthTech, Cortrium ApS, Bispebjerg Hospital, RZ Hospital, Funded By: Innovation Fund Denmark)
This project aims to enhance the assessment of Stroke and Heart Failure (HF) risk using digital biomarkers in an outpatient setting. Through a literature review, the research will identify digital markers in ECG recordings, vital signs, patient symptoms, and prior diagnoses. To address the scarcity of outpatient data, a small-scale study will be conducted for data collection. The mCardia application will be improved to gather the necessary digital biomarkers from sensors like Holter monitors and actigraphy sensors. Deep learning techniques will be employed to extract valuable parameters, including sleep quality indices, ejection fraction estimation, and blood pressure, which are crucial for risk assessment. Deep learning algorithms will be utilized to identify arrhythmias and cardiac anomalies, exploring their effects on symptoms and the risk of HF and Stroke. Additionally, an unsupervised anomaly detection module will differentiate healthy and pathologically altered ECG patterns. The project's overarching goal is to develop a reliable risk assessment framework surpassing current models for predicting Stroke and HF hospitalization or mortality, such as CHADS-VASc or Seattle Heart Failure Model.
Some of the past projects
Myo-FES: EMG-controlled FES Muscle Stimulator Device for Upper Limb Stroke Rehabilitation
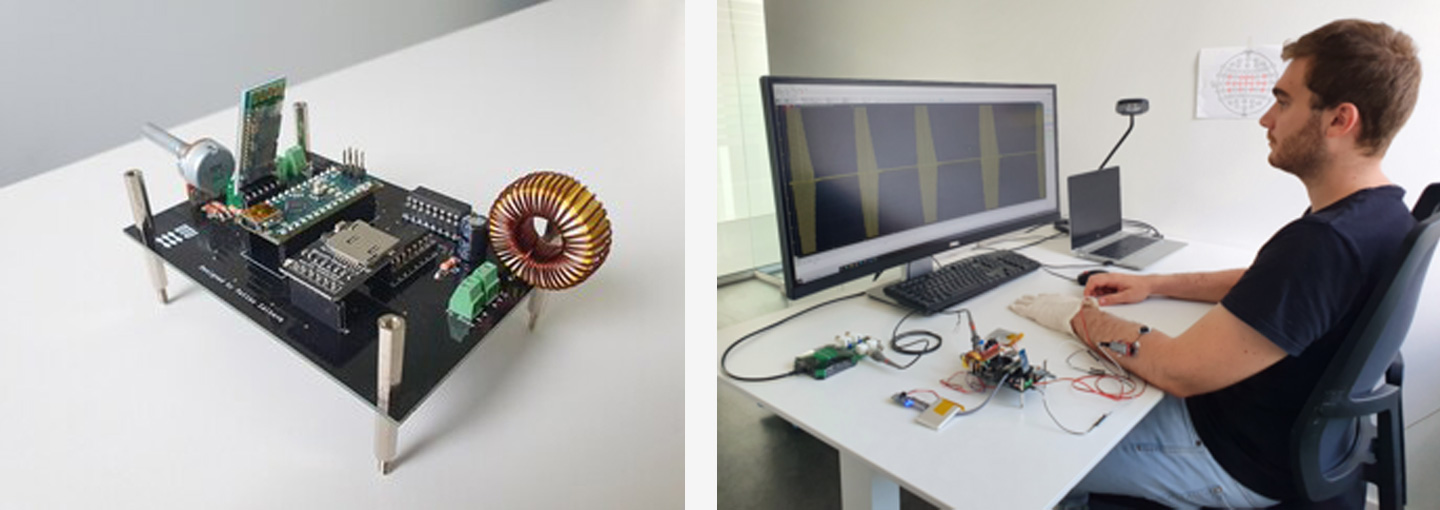
We have developed a compact, portable, and wireless EMG-controlled FES device (Myo-FES) with an embedded flex sensor for wrist extension stroke rehabilitation. This therapy takes advantage of the brain's plasticity mechanism, which allows neural pathways to be reprogrammed. Research has shown that EMG-controlled FES improves motor functions in stroke survivors with hemiparetic arm and hand. Myo-FES has two unique features: (i) It generates FES pulses of variable amplitude based on EMG signals, enabling real-time muscle activity analysis and customized electrical stimulation, and (ii) It includes strain flex sensors for continuous monitoring of wrist extension and evaluating rehabilitation progress.
EMG and Inertial Motion Sensors Based Wearable Data Acquisition System for Stroke Survivors
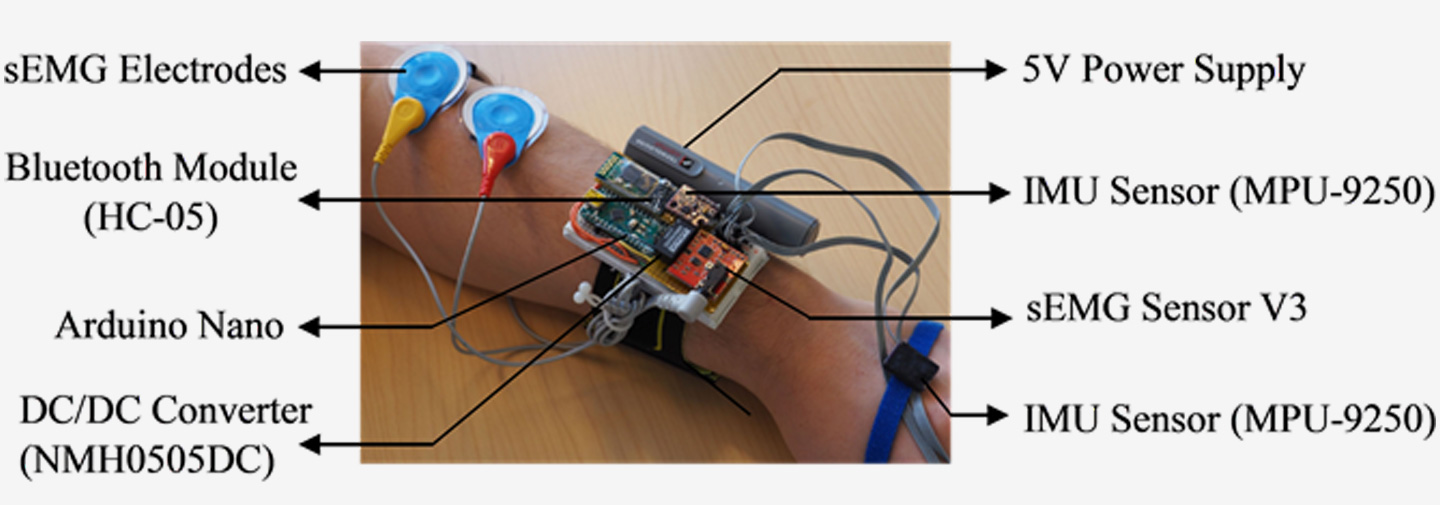
Developed a wearable data acquisition system with applications to human-machine interaction (HMI) to assist stroke survivors or people with motor disabilities. In this work, a hybrid wireless data acquisition system, which combines surface EMG (sEMG) and inertial measurement unit (IMU) sensors was built. It is designed to interface wrist extension with external devices, which allows the user to operate devices with hand orientations. A pilot study of the system performed on four healthy subjects has successfully produced two different control signals corresponding to wrist extensions. Preliminary results showed high correlation between sEMG and IMU signals, thus proving the feasibility of such a system. The generated control signals enable real-time control of various devices in daily-life activities, such as smart home lighting, electric wheelchairs, and other assistive devices. This system promotes independence by reducing dependency on caretakers and empowering individuals with disabilities to perform daily activities autonomously.
Brainy Home: A Smart Home and Wheelchair Control Application Powered by BCI
Smart home applications have become popular, in recent years, to improve the life quality of people, especially for those with motor disabilities. While the smart home applications are controlled with interaction tools such as mobile phone, voice control etc., these may not be appropriate for people with severe health issues that impacts their motor functions, for instance amyotrophic lateral sclerosis (ALS), cerebral palsy, stroke, locked-in-syndrome (LIS) etc. In this research, we have developed a smart home and wheelchair control application in a virtual environment, which is controlled solely by the steady state visual evoked potential (SSVEP) based BCI system.
Different types of BCI spellers
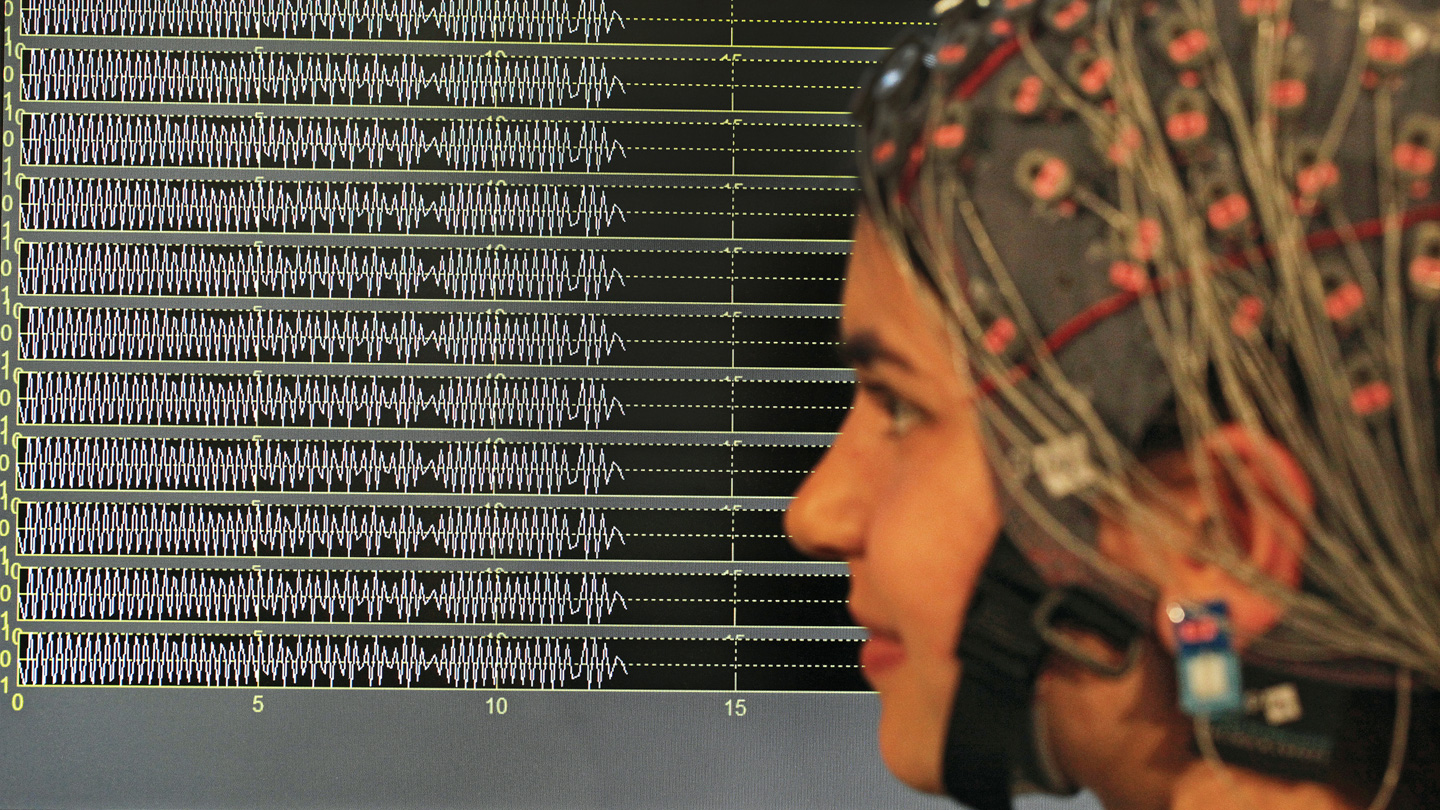
BCI spelling systems are one of the first BCI applications for individuals with lost normal communication abilities. A faster BCI spelling system with dictionary support, named as the DTU BCI Speller, which functions without any direct physical connection (eg, no mouse / keyboard) was developed in one of our earlier collaborative (DTU Elektro & Rigshospitalet) works. Once commercialized, this patented (No. WO2014207008) work, which requires only the user's visual attention, which will be a game shifter for the affected and thus, a great relief for their family.
An attempt to extend BCI speller to severely disabled individuals such as late stage ALS, a hybrid BCI spelling system combining both the gaze (DTU Management) and BCI technologies was developed. A user would only list to auditory stimuli and make simple eye movements. Successfully this recent work has great future as it allows people to communicate their intentions faster and efficiently.
Another exciting work was the development of an augmentative and alternative communication system for subjects affected by the Wolf-Hirschhorn syndrome. Their communication ability mainly relies on the assistance of a helper, who interprets the motions of their hand on a message board. This work is a step forward towards the development of an electronic aid that can replace the helper. This approach opened up an exciting path to help such disabled individuals to communicate.
BCI for locomotion
Wheelchairs are the most commonly used ADs by disabled individuals for locomotion. An attempt to help people with motor disabilities, we have developed a BCI controlled wheelchair navigation system. Simply by looking at a computer screen, the system was able to control a LEGO Mindstorm robot (wheelchair model) in 4 directions; left, right, forward and backward. The success, impact and attractiveness of this system was clearly reflected in its Danish media coverages (in MedicoTeknik, Ingeniøren, Videnskab, ALTOMTEKNIK, Medico Nyt) as well as high impact publications.
BCI for drone control
Another exciting work with DTU space was a thought controlled flying robot (Drone) to aid locked-in individuals to explore their surroundings using a video camera mounted on the drone. This stimulating work has received great attention and is being continued in our laboratory.
BCI-assisted neurorehabilitation of ADHD children
To exploit BCI's attention enhancing ability, a novel BCI system embedded within an immersive 3D virtual reality classroom was developed for neurorehabilitation of ADHD children. The system was concealed as a game with an underlying story to motivate the subjects and they could play the game simply by looking at the computer monitor. This work has received international attention, with a company from the USA (Aware Technologies) having expressed interest in this technology to use in authentic schools, learning centers and eventually in home environments.
BCI for stroke rehabilitation – Preliminary study
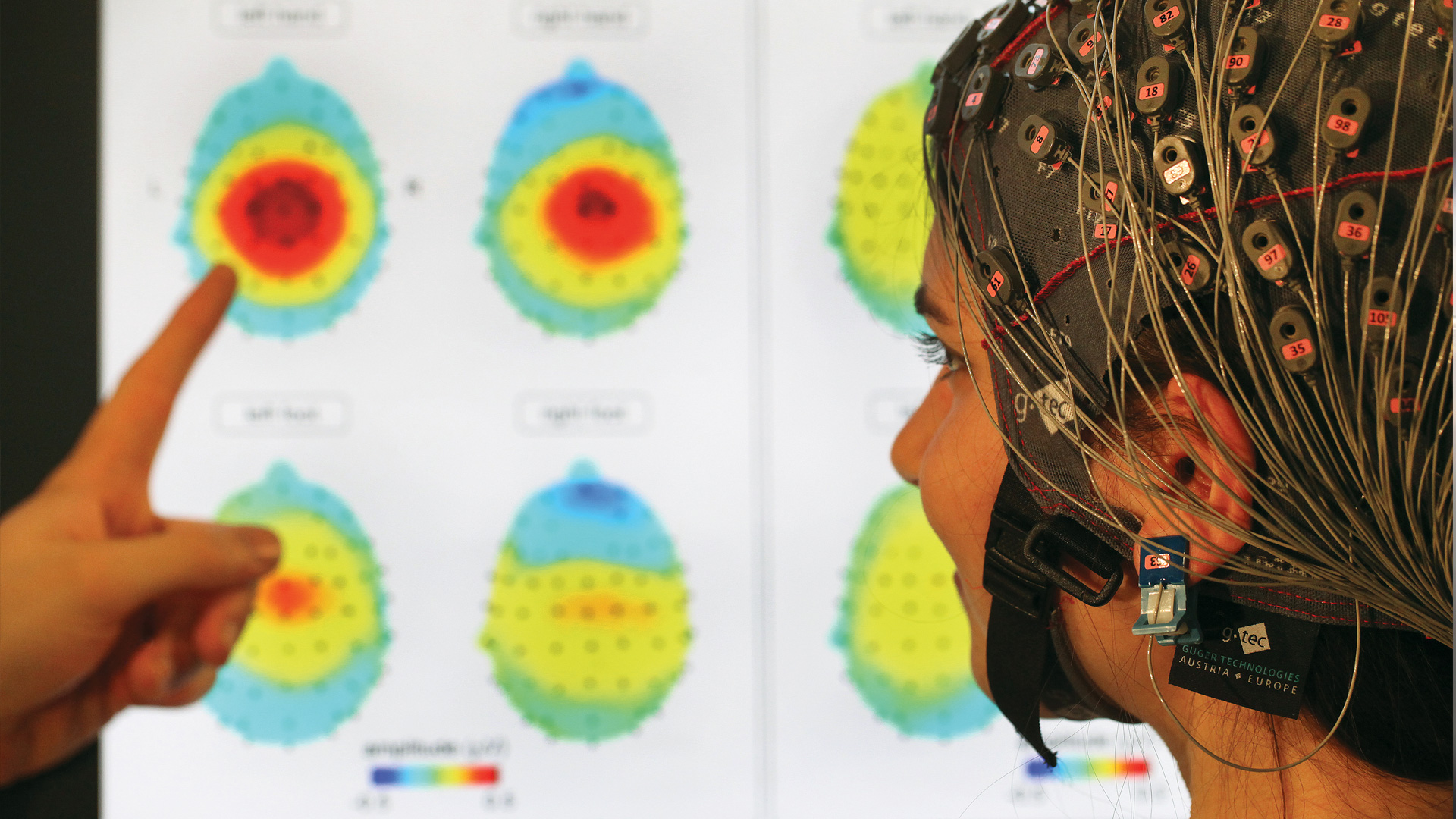
As an effort to help stroke survivors to improve their motor skills, an inexpensive rehabilitation training system is envisioned (DTU Electrical, Glostrup Hospital & Cachet). Contributions from many students to the advancement of this work have resulted in several notable publications. In recent work with DTU Management and Rigshospitalet, it has been shown that individuals could play a computer game by just thinking about moving their hands and feet. The potential impact of this for rehabilitation is very stimulating, as our results could ultimately promote rehabilitation, which is more efficient, which would result in a better recovery for millions of patients.
Neuro-stimulation for treatment of depression
An interesting collaborative (PlatoScience) work, we are aiming to develop a system (headset) capable of increasing an individual's brain activity through electrical stimulation for the treatment of depression. It is envisioned that this new product will offer a simple and effective drug-free solution, with no side effects and no technical expertise.
Ensemble deep learning for AFIB detection
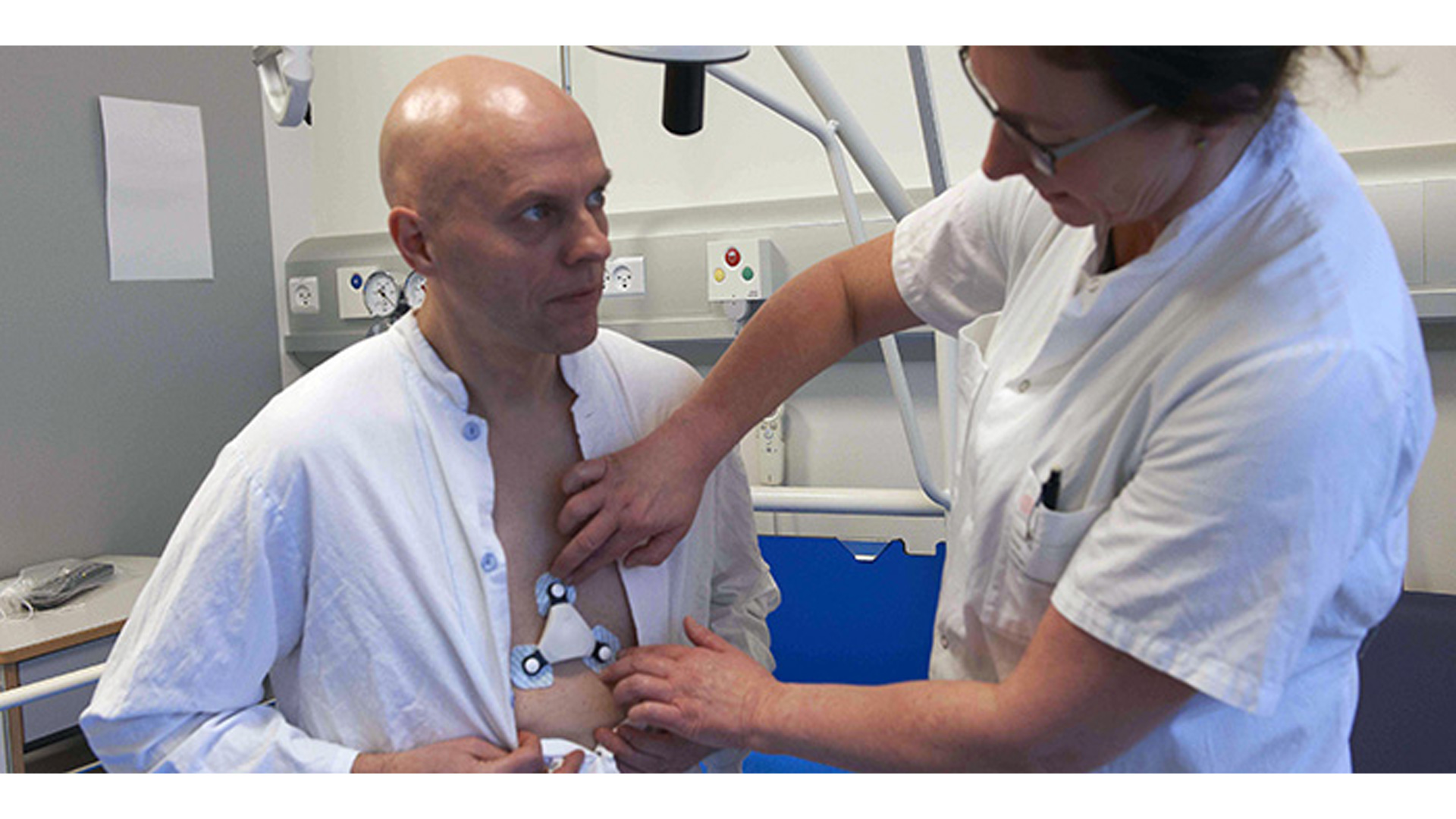
A significant proportion of worldwide mortality caused by cardiac diseases and atrial fibrillation (AFIB) is one of the most common cardiac arrhythmias among elderly population. Physicians diagnosis of AFIB through visual examination of the electrocardiogram (ECG) recordings. In our research, a novel algorithm to detect short episodes of AFIB using Machine / Deep Learning and Signal Processing methods has been developed which paves the way to extend real-time AFIB detection applications. This research (REAFEL - REAching the Frail ELderly patient for optimizing diagnosis of atrial fibrillation) is supported by the Innovation Fund Denmark (IFD Project No: 6153-00009B).
Deep Learning for Outcome Prediction in the Intensive Care Unit (ICU)
The sensors used to check the vital conditions of patients in the ICU produce huge amounts of data that can be exploited to predict the outcomes about their future conditions. In this collaborative work with Rigshospitalet, we have developed a deep learning model for the mortality prediction using the data from more than 9000 patients in different hospitals of the Capitol Region of Denmark from 2009 to 2016. Both signal processing and statistical methods are used to extract information the first 24 hours of the ICU data to build the model.
GIMIS: Gaze Input With Motor Imagery Selection
A hybrid system combining gaze and BCI for target selection in a Fitts’ Law experiment was developed. GIMIS can be used to gradually introduce BCI to late-stage ALS subjects as they begin to lose their gaze control. This work has won the Best Paper Award at COGAIN 2020.
Group Leader
Sadasivan Puthusserypady Kumaran Professor Department of Health Technology Phone: +45 45253652 sapu@dtu.dk







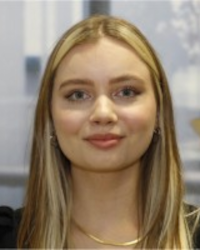









Collaborators
Jakob Eyvind Bardram Head of Sections, Professor Department of Health Technology Phone: +45 45255311 Mobile: +45 25550446 jakba@dtu.dk
Bastian Epp Groupleader, Associate Professor Department of Health Technology Phone: +45 45253953 Mobile: +45 93511485 bepp@dtu.dk
John Paulin Hansen Professor Department of Technology, Management and Economics Phone: +45 45254852 jpha@dtu.dk
Per Bækgaard Associate Professor Department of Applied Mathematics and Computer Science Phone: +45 45253908 Mobile: +45 93510543 pgba@dtu.dk
|
KIMFORD MEADOR |
|
ADA POON |
|
MAARTEN LANSBERG |
|
PREBEN KIDMOSE |
|
IRIS BRUNNER |
|
CHRISTOPH GUGER |
|
HELLE KLINGENBERG IVERSEN |
|
BALDER ONARHEIM |
|
MARIA HELENA DOMINGUEZ VALL-LAMORA |
|
ANNE FRØLICH |
|
JACOB ERIC NIELSEN |
|
ERIK SØNDERGAARD POULSEN |